Predictive maintenance: information overload, the Cassandra effect
This is the second article in a series on the hot topic of Predictive Maintenance, drawing on the practical experience of Siveco working with maintenance improvement in China and the Asia region in the past 20 years. As seen in the first article Predictions on Predictive Maintenance: it will fail… unless, ‘right data’ is better than ‘big data’. Indeed, contrary to the Internet industry, we cannot trick machines into giving away their ‘personal data’ for free… so each and every data has, practically, a cost. And how right data is, is often a matter of perspective, better framed through your maintenance strategy.
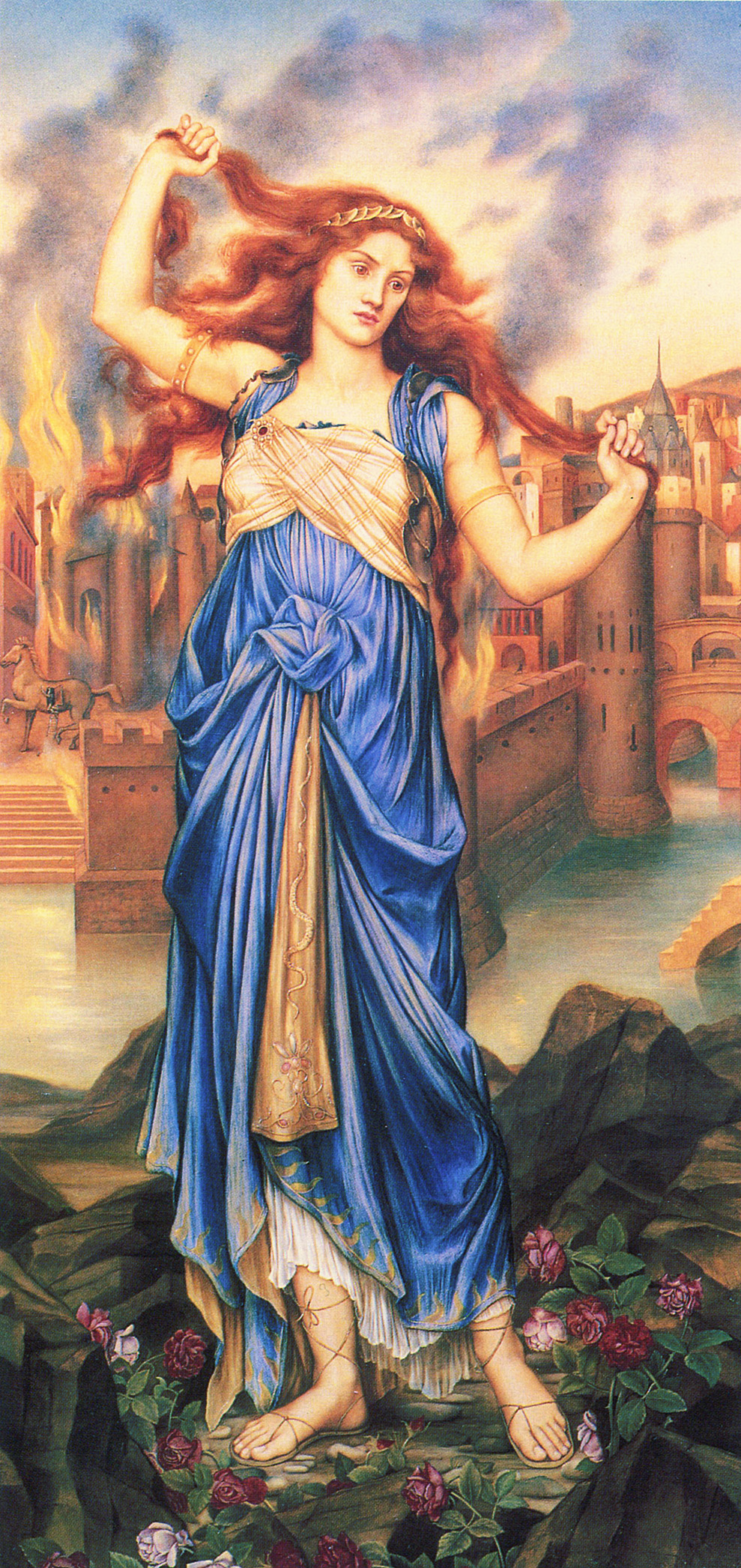
Ideally, the output of a predictive maintenance system is a notification that some system or equipment is showing early failure signs, which can be related to known failure mode and a predefined response. In most cases however, the system only provides data from sensors: alerts, measurements, meter values. Multiply these alerts and readings by the number of sensors, of machines and soon the system’s user interface looks like an illuminated Christmas tree or fireworks on New Year’s Eve. This is a classic example of information overload, also known as “the Cassandra effect”. Cassandra was a Greek mythology character who could predict the future, but nobody would believe her.
Eliminate false alarms
Contributing to information overload are the false alarms or wrong readings, resulting from sensor failure or wrong system settings… Industrial readers will recognize a familiar phenomenon sometimes observed with SCADA or BMS (Building Management Systems), with the operator’s screen filled with false. As a minimum, before transferring data to the predictive maintenance systems, false alarms, repeated alarms, should be filtered out.
Maintain the system
Sensors failure, setting errors are inevitable but a feedback loop for improvement should be put in place, so that errors are corrected over time, with a corresponding budget system maintenance. A little understood fact is that an IoT and predictive maintenance system means additional sensors, advanced technology, to be maintained by the maintenance team, increasing their burden.
Structure your data
All this data is of little value if not interpreted in relation to the maintenance strategy. Data must be organized, hierarchized based on predefined asset criticality and based on the maintenance plan. It must then be processed by the system in such a way as to support technicians in their daily jobs. Finally, it must be displayed accordingly to facilitate decisions.
Master the algorithm
A particular challenging aspect of predictive maintenance is how to ensure the organization achieves some degree of autonomy with the system. Maintenance engineers are often dependent from suppliers to adjust predictive algorithms to reflect the ever-changing challenges met by their organization. This will require qualified and trained in-house resources.
Smart O&M as enabler of predictive maintenance
All in all, many organizations still miss the intermediary steps needed to success with predictive maintenance. The Smart O&M approach advocated by Siveco, which combined asset management expertise with IT solutions, offers a comprehensive framework for the maintenance team to build a robust operating system that will process and operationalize predictive input. It involves a throughout review of the asset management and maintenance strategy in line with the ISO 55000 standard. If a predictive maintenance approach should be put in place, it can only be as part of the overall maintenance strategy. It also involved the buildup of the information system, to organize all asset and maintenance data.
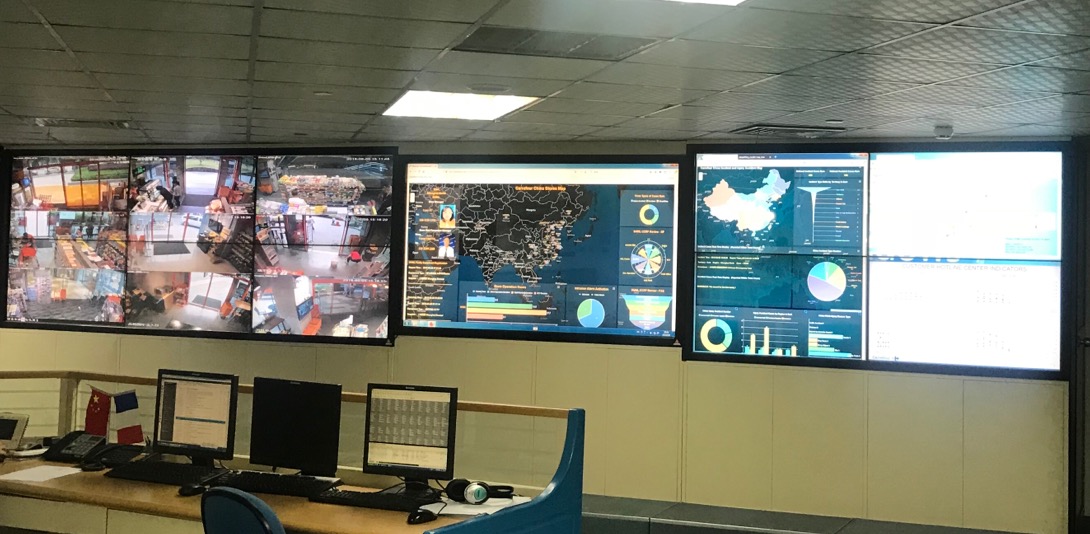
Imminent failure notifications can be channeled to the person in charge of the asset, directly to his mobile through bluebee®. Technicians can make the right decision as of when and how to treat best the alert.
In addition, historical work and failure details, including the many human inputs not covered by sensors, are available in the Smart O&M system to help nurture the predictive model, who needs accurate and structured feedback to develop and improve.
The topic of Predictive Maintenance crystallizes the dilemmas that plant managers face every day. Should they cut direct costs or to minimize consequential losses, such as downtime? Is it better to invest in new technology or to capitalize of traditional, known, methods? These questions are hard to tackle due to lack of actionable data: how to estimate consequential losses that could be avoided? How to measure the impact of a new technology? With the adoption of an ISO 55000-based approach, these apparent contradictions can be hierarchized and prioritized around the plant’s goals for safety, compliance, and reliability. Siveco proposes to leverage the Smart O&M system as a practical framework to apply ISO 55000 to address these questions, thus enabling successful Predictive Maintenance initiatives.
Our Siveco experts are frequent speakers at industry events, conferences or workshops for specific clients on the topics of Smart solutions, IoT and Predictive Maintenance. Do not hesitate to contact us at info@sivecochina.com to discuss this subject!